Data Scientist Must Know: Business x Statistics
Why integration between business and statistic is essential for Data Scientist
Why integration between business and statistic is essential for Data Scientist
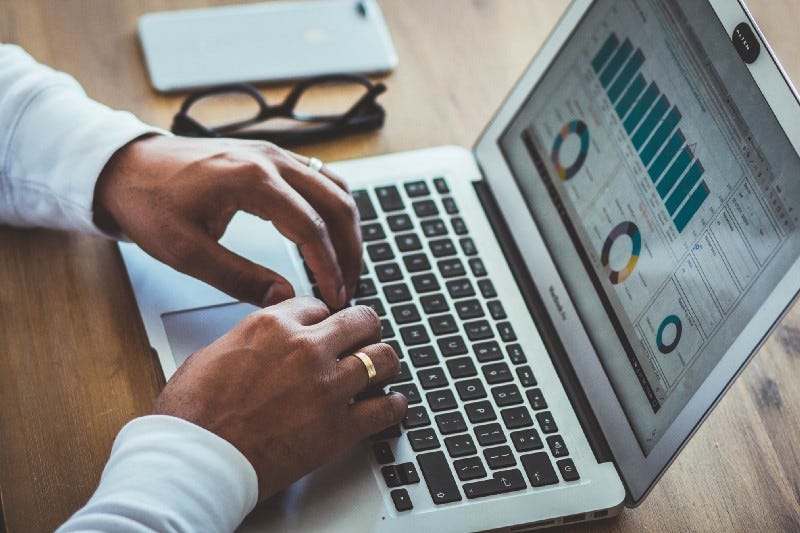
By definition, statistics is a science that deals with the collection, classification, analysis, and interpretation of data. The field is often supported by the usage of probabilistic math theory and is used to assess specific hypotheses.
The definition cannot sound more technical than it is already, and it seems business doesn’t have any to do with it. Moreover, why Data scientists need to know both of these things?
Well, statistics is more than just an advanced math class. It is the way to go for every business to get an edge from their competition. I would argue that a lot of great business leaders have made a business decision not only coming from their gut feeling but also supported by the statistic.
Any data scientist project is a data project to solve any problem the companies have. It doesn’t matter if your advance deep learning model having a 99% precision and could guess every person that passes the room; if it does not solve the business problems, it is useless.
The problem is, how exactly statistic is essential to the business and why we, as Data scientists need to understand both sides of the statistic and business? This is the question you might wonder. Well, let me explain to you a little bit more regarding business and statistics for Data Scientist.
Business x Statistic
Business and Statistic seems like two different worlds that would not merge, but it is significantly integrated. How do you ask? Let me tell you in a few passages below.
Data-Driven Bussiness and Statistic
You might often hear the term “Data-Driven” in many articles or other data science-related study material. It might say something along the line, such as “This business is data-driven,” or “The decision is based on data, so it is data-driven,” and many more.
Here, you might think that using data for your business decision means you are data-driven. Is it true? If by data, it means just looking at the number and executing the decision based on the data snapshot, then it is a
no.
Data-Driven business is more than that. The company might contain plenty of data, but if the information does not relate to the current business, it is useless.
For example, company A which has already been established for ten years wants to sell a new product for a new market. They requested their data team to profile a new market segment for their new product based on the data they have, which they claim to have a lot of data. The team then takes a look at their company data and found out by a “lot of data” means that many spreadsheet data that only contain useless attributes such as id, name, email, and phone number.
Above is an example of data that could not solve your business problem, but how about if we have a “probable” dataset to segment the new customers. Let’s say their salary, occupation, preferences, and age. Then this is a point where we need statistics in our business to evaluate the quality of the data and help the company to decide which business strategy to do.
Considering Statistic in Business
I already explain in brief why statistics is vital in the business, but what kind of statistics specifically crucial in the business. Here, we need back to the core of the business “What is the thing that matters in your business question?”; Is it the sales number, or the profit, or any kind of question you could ask. This is what we called key metrics.
For example, company A key metrics is their monthly sales number. In this case, what company A need to address is what kind of analysis they want from their key metrics. Well, The most obvious one is how the monthly sales number throughout the years. Let me give an example data below.
Now, with a simple statistic and analysis, we could see that the number of sales is increasing until February, where the sales are dropping each year immensely. In this case, statistics could help by providing the exact percentage of dropping in each year, and from a business perspective, it is worth investigating to determine the drop causes.
This is how statistic could help the business; it is not just pinpoint the problems that company have and help the business to make a business decision but also understanding what kind of the sales profile that the business has.
Business and Statistic for Data Scientist
Then, what about Data Scientist? It seems what I explained above is only applied for the business and not for Data Scientist. Well, we might need to define what Data Scientist did most of the time.
The above graph is a theoretical activity what Data Scientist do every day. While it is not wrong, the reality in the working environment is way different.
In the above graph, we could see that it is not just about cleaning and preparing data, but we need to comply with data compliance and ethic as well as to integrate any of our data projects with business problems.
Any data scientist project is a data project to solve any problem the companies have. It doesn’t matter if your advance deep learning model having a 99% precision and could guess every person that passes the room; if it does not solve the business problems, it is useless.
Every data scientist needs to understand what kind of business your company is working on and what business problem you try to solve. It is unavoidable when you are working on the company to interacting with other departments.
For example, the sales department wants to increase the number of sales. To do this, the sales team ask the data science team to create a new customer prediction model. You might think to just pull the data and train it to any machine learning model, right?
No, it is often not the case. What you need to do first is to convince the sales department would the project is viable or not and set a reasonable target. This is why data scientists need to understand the Business and the Statistic side as well.
To determine if you could execute the data project, you need useful data. In this case, you need a statistic to evaluate the quality of your data.
Also, often time, people who are not working with the data would set up an unreasonable target. For example, the sales department wants to increase the sales number by 100% next month. To prove if this target is reasonable or not, you need to evaluate it from your current data. Simple trend analysis and estimation would do the trick, but you can do this only if you understand the statistic and the business.
You might say, “Isn’t the machine learning model are created to improve the sales? If so, the estimation would be useless”. Well, the point of having a machine learning model is, of course, to solve the business problem, like increasing the sales number. As much as it is true, you still need to keep your target under a reasonable number.
There would bound a problem that your machine learning model would not foresee; for example, the resignation of the salesman, the department restructuring, distribution accident, and many more. It is great to have ambition but try to deliver your promised target in a reasonable realm.
Selecting an appropriate Key Metric could also fall to the Data Scientist’s responsibility, especially when the company is new to the data-driven business. In this case, business and statistics would become your best friend.
Conclusion
Data scientists cannot work without knowing both the Business and the Statistic. Both of these aspects are the data scientist working gear, and knowing these could give you leverage to become a better data scientist.
As a data scientist, you need to understand the business and the statistic aspect because:
Data project is not just cleaning data and creating a model, but it involves solving the business problem,
Evaluating whether the data project is viable or not to solve the business problem require statistic,
The statistic also required to have a reasonable target for your data project, because your machine learning model could not foresee everything, and
Business and statistic is a knowledge you could use to improve your position in the company or when you are applying for the data scientist position.
I hope it helps!